Artificial intelligence applied to healthcare includes a collection of technologies that enable machines to sense, interpret, act and learn. AI implementations for digital health can be relatively simple when they are focused largely on personal patient engagement, or vastly complex when working with big data sets, highly specialized diagnostics, and the workflows of multiple highly complicated organizations.
The addition of Internet of Things sensor data from connected health and related devices adds a new layer of critical, real-time contextual data. The use cases for IoT sensor-informed healthcare applications can include home security and access control for vulnerable loved ones, remote patient monitoring of vitals, activity monitoring and anomaly detection, safety of the home and mobile environments, environmental monitoring for chronic conditions, and more.
Wealth of Opportunities
These use cases provide opportunities for the application of artificial intelligence and machine learning to transform healthcare functions into data-driven services that can improve outcomes and deliver healthcare more efficiently.
Ownership of connected health devices has increased. Currently almost half (46 percent) of U.S. broadband households own at least one device, and 19 percent own at least two. Adoption of smartwatches and fitness trackers largely is responsible for driving this trend in recent years.
AI figures prominently in many of the apps that support these devices, whether it is used for applying machine learning to behavioral activity and personal health data, for supporting voice and text bot interactions, or for delivering more personalized and predictive services.
Beyond wellness uses cases, patient care applications for remote patient management, independent living, and chronic disease management offer strong AI opportunities.
Remote Patient Management and Virtual Care
Remote patient management, or RPM, may be applied to a variety of patient populations including those recently discharged after surgery, those with chronic diseases, aging adults, and other vulnerable populations.
The primary goal of RPM is to provide insight into the ongoing health status of the patient outside of clinical contexts in order to identify the need for interventions that could head off a readmission, address a decline before conditions worsen, or support better daily management of diseases.
RPM is most valuable for patients with one or more chronic conditions such as congestive heart failure, chronic obstructive pulmonary disease, coronary artery disease, diabetes, hypertension or asthma.
RPM technology typically is able to record a patient’s vital signs, activities of daily living, dietary habits, medications compliance and more. Machine learning algorithms can trigger alerts and notifications resulting in rapid interventions, significantly improved communications, better patient care, and better outcomes at lower costs than traditional healthcare delivery.
Machine learning applications also are used to train monitoring systems on massive data sets, perform predictive modeling of illness progression, and support behavioral change.
Independent Living
Twenty percent of Americans will be over the age of 65 by 2020, and the vast majority of them (84 percent) find it very important (rating 6-7 on a 7-point scale) to live independently in their own home as long as possible.
The physical and cognitive effects of aging and chronic medical conditions present challenges for those desiring to live independently, as well as for their caregivers who worry about keeping them safe, comfortable and socially connected.
With the aging demographics of the U.S. population enlarging the senior market, a growing number of companies have begun offering assistive technology that can improve seniors’ safety and wellness in the home, and enhance communication with loved ones. Many of these solutions are applying various AI applications to empower and care for aging adults.
Social engagement for an often isolated population is a key benefit provided by the service. For example, LifePod is a service that reinterprets Amazon Alexa skills to engage a senior with questions they can answer easily rather than requiring them to know the precise wording Amazon Skills typically require.
By fusing data from a range of sensors, the application can track where a person is in the residence, how long they remain in a particular spot, or how many meals they eat and at what time. It also tracks and analyzes a person’s gait, factors that could lead to fall risk, and daily activities including personal hygiene and sleeping patterns.
This tracking leads to personalized care by building a contextual understanding of each person’s normal daily activities, and uncovers potential risks to help minimize hospital readmission rates.
These types of applications apply machine learning and predictive analytics to data gathered by smart home sensors, security systems, connected medical devices, health and fitness wearables, personal emergency response systems (PERS), and smart speakers.
The machine learning application in turn translates the data into behavioral activities to create a profile of normal behavior for each customer. As the system compares daily activities to the stored model, it can identify anomalies and issue alerts for trends that are not normal or healthy.
The business model for independent living solutions largely still follows the private pay subscription model, but both seniors and their caregivers indicate a strong interest in paying for these services. Fifty percent of older consumers (ages 50-plus) report being very interested in an emergency panic button service or home emergency system.
The majority (58 percent) of older consumers also are willing to pay $30/month for an independent living system that has all of their must-have features. New business-to-business opportunities are emerging for independent living solutions customized for senior living facilities and communities.
Chronic Disease Management
The proliferation of smartphones and connected medical devices provides new opportunities for helping those living with chronic conditions to be monitored and remotely supported for improved health and safety.
Fifty-seven percent of consumers in U.S. broadband households report at least one chronic condition, and 28 percent have two or more.
Robust solutions are coming to market for supporting patients with diabetes, heart disease, obesity and sleep disorders.
As with other AI tools, machine learning applications for chronic disease management are first trained on large datasets of specific populations to identify patterns of disease progression and behavioral patterns that drive changes in health status.
Data from personal medical devices, smart sensors and electronic health records concerning behavioral activities such as diet, exercise and stress levels can be fused to uncover insights into why a patient’s health status may change. Prediction and recommendation engines can inform behavioral change engagements through apps and other interfaces.
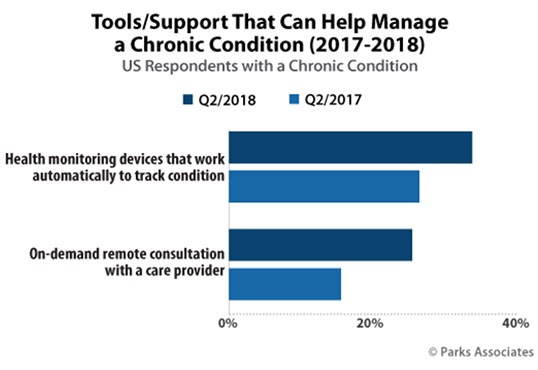
The high costs of managing chronic conditions are driving healthcare companies to invest and innovate using AI technologies. Strong ecosystems are developing among sensor and device makers, platform providers, data science software developers, user experience experts, and behavioral scientists, providers and payers.
Monitoring behavioral change is a difficult and complex task, but the personalized experiences enabled by AI technologies offer promising new tools for engaging patients every day of their lives.
Hello Russell!!! I AM so glad by going through this article. It is a very wonderful thing that we can implement the AI in the directions of healthcare by including a collection of technologies. As you mentioned AI implementations for digital health can be relatively simple when they are focused largely on personal patient engagement, or vastly complex when working with big data sets, highly specialized diagnostics, and the workflows of multiple highly complicated organizations.