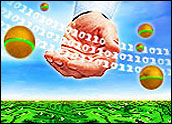
Will 2009 see the redemption of the risk management profession, or is the renewed focus on risk a case of locking the stable doors after the horse has bolted? There is tremendous pressure from clients, regulators and politicians to get it right and invest in risk management practices that will prevent a repeat of events. As banks reassess current risk management techniques and supporting infrastructure, budget cuts and pressure to move quickly make it harder to step back and look at the challenge not only from the top down but from the bottom up.
Any risk system is only going to be as good as the data it relies on. The drive to remove data silos and create golden copy master data that can be accessed from across the organization, ensuring a single consistent and up-to-the minute view, is more important than ever. While this might seem overwhelming, the good news is that there are new enterprise data management tools and best practices that can transform the grim outlook for the risk management community.
Clean Data Is Good Data
Data can never be too clean or too fast. There is a clear and pressing need for risk managers to have access to more timely data. They need to be able to establish linkages across data types, increase depth of information behind investments, and build flexibility and support for various hierarchies of risk measures in data systems. But before the data can be put to work, risk managers will need to ensure that they have clean, complete and timely data to start with.
Risk managers realize that data is of fundamental importance and must act now to execute a credible and pragmatic data management strategy. They need to consider how good the enterprise risk architecture is at absorbing and disseminating data in different formats. Often, the proprietary and closed nature of risk applications’ data models means it is impossible to support actions and products which are not “native” to any specific risk engine’s data model. When critical data is held in proprietary databases, risk managers lose the opportunity to utilize that data across business boundaries, creating a fragmented and siloed data landscape. Once data is centralized in a single repository, any links, hierarchies and other relationships that may exist between products, issuers, customers and counterparties become fully transparent to risk managers and accessible from multiple risk, finance and audit applications.
There are also many technological barriers to a more integrated data infrastructure. Risk analytics engines demand a baffling diversity of middleware, data import/export and interfaces. This means that many organizations first pick the risk engine they will use and then interface back into the required data environment for the engine. Developing a timely, accurate and consolidated data infrastructure for risk engines and managers to draw from is a more robust method.
It is not feasible for an institution to run separate data management systems for each function that needs data. Data management must become an enterprise-wide strategic function which can run risk analysis, accounting, operations research and other critical functions.
Unrealized Truth in Consolidated Data
In many banks, important risk-relevant data is scattered through the organization within different systems, on pieces of paper in multiple buildings or in different formats within one system. It is important to align the strategic data management programs of the company as a whole when creating data collection and systems initiatives.
Too often data is kept without reference to the links and relationships which can turn it into actionable information. Data from different operational silos can also be left unconsolidated. Instead of working from a common data source, collecting and analyzing data on a unit-by-unit basis can expose the business to credit and operational risk.
The process usually starts with the sourcing of data from market data vendors like Bloomberg, Thomson/Reuters and others. The acquisition of market and reference data has its own challenges. Every data provider uses different metadata formats which must be integrated with internal systems. This will allow data to retain its accuracy while being aggregated to the enterprise level and create a golden copy of the data.
The situation grows more complex once external market data sources are integrated, and this external data is combined with internal information. To develop mark-to-market reporting requirements and produce consistent flows of data usable in accounts and general ledger work, external market data must be consistently compared and combined with internal data.
Additional technology-induced operational risks are created when banks’ data management capabilities trail significantly behind operations. While these risks can be avoided, organizations must take a proactive approach by implementing an intelligent and strategically focused data management system.
Time Constraints
Ideally, funding and risk would be managed through real-time consolidation of all current and anticipated cash and positions, and then integrated with accurate market and counterparty risk data. Maintaining a real-time stream of clean and accurate data, derived from multiple disparate systems, is the only way to close the gap between actual and projected liquidity and risk exposure.
Identifying counterparty risk is only the latest high-profile use for enterprise data management. Real-time counterparty exposure management remains a priority for most firms, even though many are relying on manual efforts or out-of-date sources. Linking counterparty risk data to transactions and positions can take hours or days. For many risk scenarios, this is too little, too late.
Michael Meriton is CEO of enterprise data management firm GoldenSource.
Social Media
See all Social Media